Christopher A. Choquette-Choo
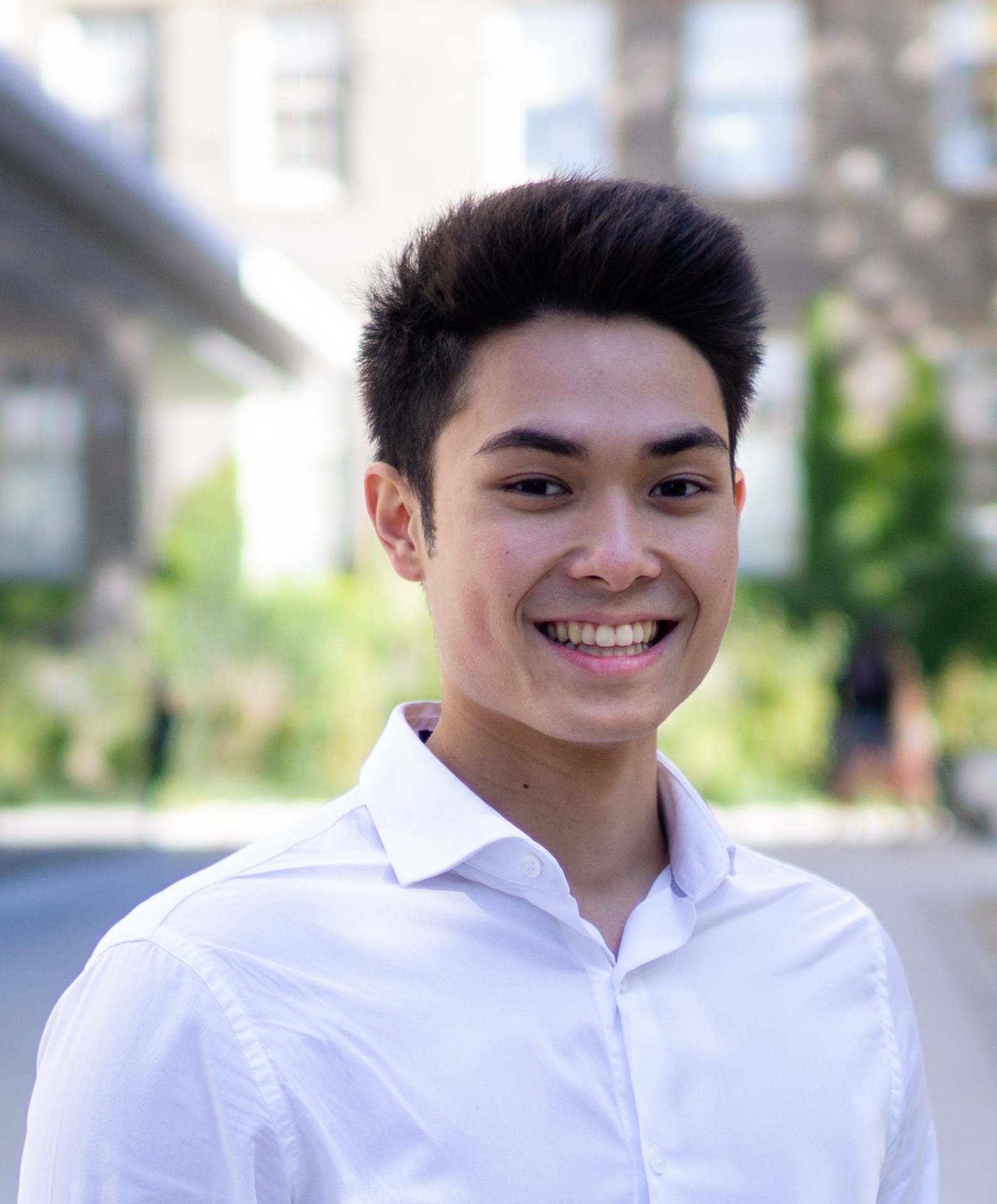
I am a Research Scientist in Google Deepmind, on the DeepMind Privacy and Security team working on fundamental and applied research. I lead privacy evals, among other contributions, for our frontier model efforts, including Gemini (+1.5 Pro/Flash), Gemma (+CodeGemma), PaLM 2, and GBoard. My work has ensured we meet compliance, enabling model releases.
Previously, I was an AI Resident at Google and a researcher in the CleverHans Lab at the Vector Institute. I graduated from the University of Toronto, where I had a full scholarship.
Email: choquette[dot]christopher[at]gmail[dot]com
Research
My focuses are privacy-preserving and adversarial machine learning. I mainly work on studying memorization and harms in language modelling as well as improving DP algorithms for machine learning; but, I've also worked on privacy auditing techniques, collaborative learning approaches, and methods for ownership-verification.
See my google scholar for an up-to-date list.
Technical Reports and Production Deployments |
|
Milad Nasr, Nicholas Carlini, Jonathan Hayase, Matthew Jagielski, A. Feder Cooper, Daphne Ippolito, Christopher A. Choquette-Choo, Eric Wallace, Florian Tramèr, Katherine Lee preprint We show that production aligned models can be provide a false-sense of privacy.We show a vulnerability in Chat-GPT that lets us extract a lot of data. We scalably test for extractable memorization in many open-source models. |
|
Christopher A. Choquette-Choo, Arun Ganesh, Thomas Steinke, Abhradeep Guha Thakurta International Conference on Learning Representations (ICLR), 2024conference (+spotlight) We prove a generic privacy amplification technique for DP-FTRL. |
|
Nikhil Kandpal, Krishna Pillutla, Alina Oprea, Peter Kairouz, Christopher A. Choquette-Choo, Zheng Xu Socially Responsible Language Modelling Research (SoLaR), 2023workshop International Workshop on Federated Learning in the Age of Foundation Models in Conjunction with NeurIPS (FL@FM-NeurIPS), 2023workshop The first data-source level inference attacks on LLMs. We can detect if a particular user's was used to train a model with high success even with few documents and without any actualy training documents from a user. |
|
Harsh Chaudhari, Giorgio Severi, John Abascal, Matthew Jagielski, Christopher A. Choquette-Choo, Milad Nasr, Cristina Nita-Rotaru, Alina Oprea preprint New security and privacy against RAG models. |
|
USVSN Sai Prashanth, Alvin Deng, Kyle O'Brien, Jyothir S V, Mohammad Aflah Khan, Jaydeep Borkar, Christopher A. Choquette-Choo, Jacob Ray Fuehne, Stella Biderman, Tracy Ke, Katherine Lee, Naomi Saphra preprint We create a taxomonomy describing memorization in LLMs. |
|
Christopher A. Choquette-Choo, Arun Ganesh, Abhradeep Thakurta preprint New optimal rates for stochastic convex optimization with differential privacy. |
|
Christopher A. Choquette-Choo, Krishnamurthy Dj Dvijotham, Krishna Pillutla, Arun Ganesh, Thomas Steinke, Abhradeep Guha Thakurta International Conference on Learning Representations (ICLR), 2024conference International Workshop on Federated Learning in the Age of Foundation Models (FL@FM-NeurIPS)2023workshop We prove bounds on DP-FTRL that show when it is better than DP-SGD. We design new algorithms that are more efficient and as performant. |
|
Ashwinee Panda, Christopher A. Choquette-Choo, Zhengming Zhang, Yaoqing Yang, Prateek Mittal International Conference on Learning Representations (ICLR), 2024conference We show new attacks that can extract sensitive PII from LLMs with high success and minimal assumptions. |
|
Edoardo Debenedetti, Giorgio Severi, Milad Nasr, Christopher A. Choquette-Choo, Matthew Jagielski, Eric Wallace, Nicholas Carlini, Florian Tramèr Proceedings of 33th USENIX Security, 2024conference We show that an entire system---not just an ML model---need to be considered to ensure privacy. We introduce new attacks against a variety of systems components that can invalidate guarantees on just an ML model. |
|
A. Feder Cooper*, Katherine Lee*, James Grimmelmann, Daphne Ippolito, Christopher Callison-Burch, Christopher A. Choquette-Choo, ... preprint We discuss and provide insights/opinions on the intersection of generative AI and law. |
|
Sneha Kudugunta, Isaac Caswell, Biao Zhang, Xavier Garcia, Christopher A. Choquette-Choo, Katherine Lee, Derrick Xin, Aditya Kusupati, Romi Stella, Ankur Bapna, Orhan Firat Thirty-seventh Conference on Neural Information Processing Systems (Neurips), 2023conference We introduce a new multilingual dataset, improve multilingual models, and show that translation tasks can be vulnerable to new privacy attacks. |
|
![]() |
Christopher A. Choquette-Choo, H. Brendan McMahan, Keith Rush, Abhradeep Thakurta 40th International Conference on Machine Learning (ICML), 2023conference (+oral) We achieve new state-of-the-art privacy-utility tradeoffs in DP ML. We outperform DP-SGD without any need for privacy amplification. |
![]() |
Enayat Ullah*, Christopher A. Choquette-Choo*, Peter Kairouz*, Sewoong Oh* 40th International Conference on Machine Learning (ICML), 2023conference We show how to achieve impressive compression rates in the online setting, i.e., without prior tuning. Our best algorithms can achieve close to the best-known compression rates. |
![]() |
Christopher A. Choquette-Choo, Arun Ganesh, Ryan McKenna, H. Brendan McMahan, Keith Rush, Abhradeep Guha Thakurta, Zheng Xu Thirty-seventh Conference on Neural Information Processing Systems (Neurips), 2023conference We show that DP-FTRL is strictly better than DP-SGD. In doing so, we get new state-of-the-art. |
Nicholas Carlini, Milad Nasr, Christopher A. Choquette-Choo, Matthew Jagielski, Irena Gao, Anas Awadalla, Pang Wei Koh, Daphne Ippolito, Katherine Lee, Florian Tramèr, Ludwig Schmidt Thirty-seventh Conference on Neural Information Processing Systems (Neurips), 2023conference We introduce attacks against multi-modal models that subvert alignment techniques. In doing so, we show that current NLP attacks are not sufficiently powerful to evaluate adversarial alignment. |
|
Nicholas Carlini, Matthew Jagielski, Christopher A. Choquette-Choo, Daniel Paleka, Will Pearce, Hyrum Anderson, Andreas Terzis, Kurt Thomas, Florian Tramèr IEEE Symposium on Security and Privacy (IEEE S&P), 2024conference We show that modern large-scale datasets can be cost-effectively poisoned. |
|
![]() |
Matthew Jagielski, Milad Nasr, Katherine Lee, Christopher A. Choquette-Choo, Nicholas Carlini conference (+oral) We show that, without formal guarantees, distillation provides limited privacy benefits. We also show the first attack where a model can leak information about private data without ever having been queried on that private data. |
![]() |
Daphne Ippolito, Florian Tramèr*, Milad Nasr*, Chiyuan Zhang*, Matthew Jagielski*, Katherine Lee*, Christopher A. Choquette-Choo*, Nicholas Carlini * Equal contribution. The names are ordered randomly. Proceedings of the 15th International Natural Language Generation Conference (INLG) 2023conference (best-paper runner-up) We show that models can paraphrase memorizations. Thus, models can evade filters designed specifically to prevent verbatim memorization, like those implemented for CoPilot. |
Karan Chadha, Matthew Jagielski, Nicolas Papernot, Christopher A. Choquette-Choo, Milad Nasr International Conference on Machine Learning (ICML), 2024 conference |
|
Congyu Fang*, Hengrui Jia*, Anvith Thudi, Mohammad Yaghini, Christopher A. Choquette-Choo, Natalie Dullerud, Varun Chandrasekaran, Nicolas Papernot * Equal contribution. Proceedings of the 8th IEEE Euro S&P 2023conference We break current Proof-of-Learning schemes. Though we design schemes that are robust to all current attacks, we show that it is an open problem to provide formal guarantees. |
|
Nicholas Franzese, Adam Dziedzic, Christopher A. Choquette-Choo, Mark R. Thomas, Muhammad Ahmad Kaleem, Stephan Rabanser, Congyu Fang, Somesh Jha, Nicolas Papernot, Xiao Wang Thirty-seventh Conference on Neural Information Processing Systems (Neurips) 2023conference
|
|
Adam Dziedzic, Christopher A. Choquette-Choo, Natalie Dullerud, Vinith Menon Suriyakumar, Ali Shahin Shamsabadi, Muhammad Ahmad Kaleem, Somesh Jha, Nicolas Papernot, Xiao Wang Proceedings on 23rd Privacy Enhancing Technologies Symposium (PETS 2023)conference
|
|
Yannis Cattan, Christopher A Choquette-Choo, Nicolas Papernot, Abhradeep Thakurta preprint Do proper hyper-parameter tuning with DP. Check how many layers you should tune. |
|
![]() |
Wei-Ning Chen*, Christopher A. Choquette-Choo*, Peter Kairouz*, Ananda Theertha Suresh* * Equal contribution. The names are ordered alphabetically. International Conference on Machine Learning (ICML), 2022 conference (+spotlight) Theory and Practice of Differential Privacy (TPDP) Workshop, 2022 workshop We characterize the fundamental communication costs of Federated Learning (FL) under Secure Aggregation (SecAgg) and Differential Privacy (DP), two privacy-preserving mechanisms that are commonly used with FL. We prove our optimality for worst-case settings which provides significant improvements over prior work, and show that improvements can be made under additional assumptions, e.g., data sparisty. Extensive empirical evaluations support our claims, showing costs as low as 1.2 bits per parameter on Stack Overflow with <4% relative decrease in test-time model accuracy. |
![]() |
Wei-Ning Chen*, Christopher A. Choquette-Choo*, Peter Kairouz* Privacy in Machine Learning Workshop at Neurips, 2021 workshop * Equal contribution. The names are ordered alphabetically. We show that in the worst-case, differentially-private federated learning with secure aggregation requires Ω(d) bits. Despite this, we discuss how to leverage near-sparsity to compress updates by more than 50x with modest noise multipliers of 0.4 by using sketching. |
Hengrui Jia^, Mohammad Yaghini^, Christopher A. Choquette-Choo*, Natalie Dullerud*, Anvith Thudi*, Varun Chandrasekaran, Nicolas Papernot Proceedings of the 42nd IEEE Symposium on Security and Privacy, San Francisco, CA, 2021 conference ^,* Equal contribution. The names are ordered alphabetically. How can we prove that a machine learning model owner trained their model? We define the problem of Proof-of-Learning (PoL) in machine learning and provide a method for it that is robust to several spoofing attacks. This protocol enables model ownership verification and robustness against byzantines workers (in a distributed learning setting). |
|
![]() |
Christopher A. Choquette-Choo*, Natalie Dullerud*, Adam Dziedzic*, Yunxiang Zhang*, Somesh Jha, Nicolas Papernot, Xiao Wang 9th International Conference on Learning Representations (ICLR), 2021 conference * Equal contribution. The names are ordered alphabetically. We design a protocol for collaborative learning that ensures both the privacy of the training data and confidentiality of the test data in a collaborative setup. Our protocol can provide several percentage-points improvement to models, especially on subpopulations that the model underperforms on, with a modest privacy budget usage of less than 20. Unlike prior work, we enable collaborative learning of heterogeneous models amongst participants. Unlike differentially private federated learning, which requires ~1 million participants, our protocol can work in regimes of ~100 participants. |
![]() |
Christopher A. Choquette-Choo, Florian Tramèr, Nicholas Carlini, Nicolas Papernot 38th International Conference on Machine Learning (ICML), 2021 conference (+spotlight) What defenses properly defend against all membership inference threats? We expose and show that confidence-masking -- defensive obfuscation of confidence-vectors -- is not a viable defense to Membership Inference. We do this by introducing (3) label-only attacks, which bypass this defense and match typical confidence-vector attacks. In an extensive evaluation of defenses,including the first evaluation of data augmentations and transfer learning as defenses, we further show that Differential Privacy can defend against average- and worse-case Membership Inference attacks. |
![]() |
Hengrui Jia, Christopher A. Choquette-Choo, Varun Chandrasekaran, Nicolas Papernot Proceedings of 30th USENIX Security, 2021 conference How can we enable an IP owner to reliably claim ownership of a stolen model? We explore entangling of watermarks to task data to ensure that stolen models learn these watermarks as well. Our improved watermarks enable IP owners to claim ownership with 95% confidence in less than 10 queries to the stolen model. |
![]() |
Lucas Bourtoule*, Varun Chandrasekaran*, Christopher A. Choquette-Choo*, Hengrui Jia*, Adelin Travers*, Baiwu Zhang*, David Lie, Nicolas Papernot Proceedings of the 42nd IEEE Symposium on Security and Privacy, San Francisco, CA, 2021 conference * Equal contribution. The names are ordered alphabetically. How can we enable efficient and guaranteed retraining of machine learning models? We define requirements for machine unlearning and study a stricter unlearning requirement whereby unlearning a datapoint is guaranteed to be equivalent to as if we had never trained on it. To this end, we improve on the naive retraining-from-scratch approach to provide a better accuracy-efficieny tradeoff. We also study how a priori knowledge of the distribution of requests can further improve efficiency. |
![]() |
Christopher A. Choquette-Choo, David Sheldon, Jonny Proppe, John Alphonso-Gibbs, Harsha Gupta ICMLA, 2019  |  DOI: 10.1109/ICMLA.2019.00161 conference How can we triage bugs more effectively? By utilizing a model's own knowledge of an analogous lower-dimensionality solution-space (predicting the correct team assignment), we can achieve higher accuracies on the higher-dimensionality solution-space (predicting the correct engineer assignment). |
Paper Talks
DP-Follow-The-Regularized-Leader: State-of-the-art Optimizers for Private Machine Learning.
Invited talk at Institute of Science and Technology Austria (ISTA) for Prof. Christoph Lampert in 2024. |
DP-Follow-The-Regularized-Leader: State-of-the-art Optimizers for Private Machine Learning.
Invited talk at the “Federated Learning on the Edge” AAAI Spring 2024 Symposium. |
Hosted the "Private Optimization with Correlated Noise" invited session. Co-presented first talk.
Presented at Information Theory and Applications (ITA) 2024. |
Poisoning Web-Scale Training Datasets is Practical.
Presented in lecture for Prof. Varun Chandrasekaran at University of Illinois at Urbana-Champaign in 2024. |
Multi-Epoch Matrix Factorization Mechanisms for Private Machine Learning.
Presented at ICML 2023 ICML Website or SlidesLive archive |
The Fundamental Price of Secure Aggregation in Differentially Private Machine Learning
Invited talk at the University of Toronto & Vector Institute for Prof. Nicolas Papernot. ICML Website |
The Fundamental Price of Secure Aggregation in Differentially Private Machine Learning
Presented at ICML 2022 ICML Website |
Label-Only Membership Inference Attacks
Presented at ICML 2021 ICML Website |
Proof-of-Learning Definitions and Practices
Presented at IEEE S&P 2021 S&P Youtube Channel |
Machine Unlearning
Presented at IEEE S&P 2021 S&P Youtube Channel |
Invited Talks
![]() |
Privacy Considerations of Production Machine Learning.
Presented at Ml Ops World: New York Area Summit Contact me for slides. Unfortunately, the video is not publicly available :(. |
![]() |
Adversarial Machine Learning: Ensuring Security and Privacy of ML Models and Sensitive Data.
Presented at the REWORK Responsible AI Summit 2019. Available as a part of the Privacy and Security in Machine Learning package. |
Professional Services
- AC for the 2024 Thirty-eighth Neural Information Processing Systems (Neurips).
- PC for the 2025 IEEE Security and Privacy (S&P) conference.
- Reviewer for the 2024 Forty-first International Conference on Machine Learning (ICML).
- Reviewer for the 2024 Twelfth International Conference on Learning Representations (ICLR).
- PC for the 2024 IEEE Security and Privacy (S&P) conference.
- PC for the 2023 Generative AI + Law (GenLaw)'23 Workshop at ICML
- Top Reviewer for the 2023 Neural Information Processing Systems (Neurips).
- Reviewer for 2023 International Conference on Machine Learning (ICML)
- Invited Reviewer for Nature Machine Intelligence Journal 2023
- Session Chair of DL: Robustness for the 2022 International Conference on Machine Learning (ICML).
- Reviewer for the 2022 Neural Information Processing Systems (Neurips).
- Invited Reviewer for Nature Machine Intelligence Journal 2022
- Outstanding Reviewer for 2022 International Conference on Machine Learning (ICML)
- Invited Reviewer for 2022 IEEE Transactions on Emerging Topics in Computing
- External Reviewer for 2022 USENIX Security Symposium
- External Reviewer for 2022 IEEE Symposium on Security and Privacy
- Reviewer for 2021 Journal of Machine Learning Research
- External Reviewer for 2021 International Conference on Machine Learning (ICML)
- External Reviewer for 2021 USENIX Security Symposium
- External Reviewer for 2021 IEEE Symposium on Security and Privacy
- Reviewer for the 2020 Machine Learning for the Developing World (ML4D) workshop at NeurIPS